Essential Skills for Success in Data Analysis and Computational Science Careers
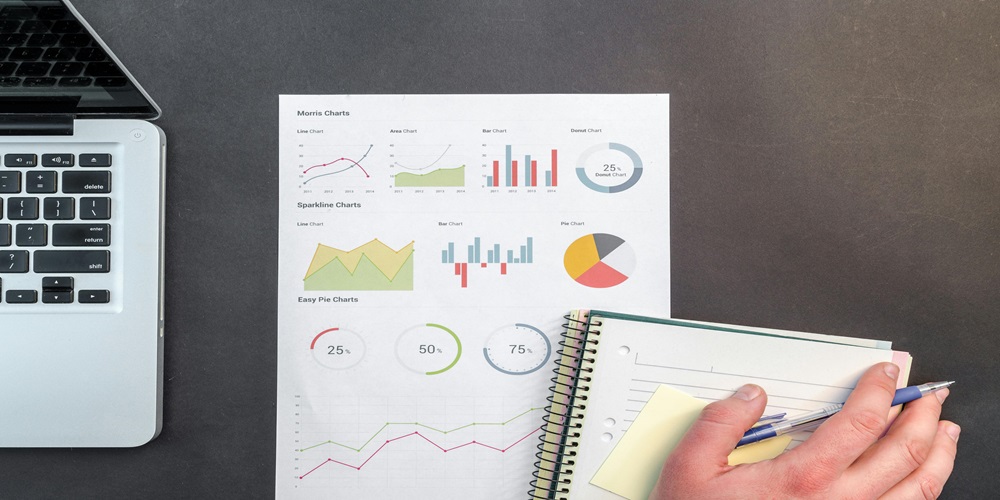
Whether you’re eyeing a career in data analysis or computational science, mastering the right skills is crucial for standing out in this competitive field. Imagine wielding incredible tools and techniques that not only enhance your decision-making but also empower businesses to thrive in an era of rapid technological advancement! In this blog post, we’ll explore the essential skills you need to succeed—ranging from technical expertise with programming languages like Python and R to critical thinking and effective communication strategies. Get ready to embark on a journey that will equip you with the knowledge and confidence needed to excel in your chosen career path! Let’s dive into what it takes to become a powerhouse in data analysis and computational science!
The growing field of Data Analysis and Computational Science
Organizations are inundated with vast amounts of data, and they need skilled professionals to make sense of it all. This growing field not only offers exciting career opportunities but also presents a chance to drive meaningful change across industries.
As businesses turn to data-driven decision-making, the importance of acquiring essential skills in this domain cannot be overstated. Whether you’re just starting your journey or looking to elevate your existing skill set, understanding what it takes to succeed in data analysis and computational science careers is crucial. Let’s dive into the key skills you need to thrive in this dynamic environment!
Importance of acquiring essential skills in this field for career success
The landscape of Data Analysis and Computational Science is evolving rapidly. With the explosion of data across industries, individuals equipped with essential skills stand out in a crowded job market.
Employers are no longer just looking for candidates who can crunch numbers. They seek professionals who can interpret complex datasets and translate findings into actionable insights. This ability drives strategic decision-making in businesses today.
Moreover, acquiring these skills isn’t merely about securing a job; it’s about being adaptable to changing technologies and methodologies. As tools evolve, so do the techniques required to harness their power effectively.
Investing time in skill development not only enhances employability but also prepares you for leadership roles down the line. It fosters confidence and creativity—qualities that set successful professionals apart from their peers in this dynamic field.
Key skills required for Data Analysis and Computational Science careers
Data Analysis and Computational Science careers demand a blend of technical and non-technical skills.
On the technical side, proficiency in programming languages like Python, R, and SQL is crucial. These languages form the backbone of data manipulation and analysis tasks. Mastery in statistical analysis enables professionals to interpret complex datasets effectively.
Machine learning is another vital area. Understanding algorithms helps in making predictive models that can drive business strategies.
Equally important are non-technical skills. Strong communication abilities allow you to convey findings clearly to stakeholders who may not be familiar with data jargon. Problem-solving agility ensures you can navigate challenges creatively as they arise.
Critical thinking plays a key role too; it empowers analysts to evaluate information from various angles before making decisions or recommendations. Each skill complements the others, crafting well-rounded candidates for Data Analysis and Computational Science jobs.
Technical skills: Programming languages (Python, R, SQL), Statistical analysis, Machine learning
Technical skills form the backbone of a successful career in Data Analysis and Computational Science.
Programming languages like Python, R, and SQL are essential tools for any data professional. Python’s versatility makes it popular for various applications, from web development to machine learning. R is tailored specifically for statistical analysis, providing robust libraries that simplify complex computations.
SQL serves as the standard language for managing databases effectively. Mastering these three languages opens doors to endless possibilities in data manipulation and analysis.
Statistical analysis is crucial too. It allows you to interpret data trends accurately and make informed decisions based on quantitative evidence. Understanding concepts such as regression models or hypothesis testing enriches your analytical capabilities.
Machine learning adds another layer of depth to your skill set. This field enables you to create algorithms that can learn from data patterns over time, driving innovation across industries. Embracing these technical skills will set you apart in the competitive job market.
Non-technical skills: Communication, Problem-solving, Critical thinking
Non-technical skills play a pivotal role in the realm of Data Analysis and Computational Science Jobs. Communication is essential, as analysts must convey complex findings to diverse audiences. The ability to translate data into actionable insights can set candidates apart.
Problem-solving skills are equally crucial. Analysts face various challenges that require innovative solutions. A sharp mind can dissect issues and identify patterns that may not be immediately obvious.
Critical thinking empowers professionals to evaluate information rigorously. This skill aids in assessing data validity and making informed decisions based on evidence rather than assumptions.
Cultivating these non-technical abilities enhances collaboration within teams, allowing for diverse ideas to flourish while driving projects forward effectively. Ultimately, mastering these soft skills creates a balanced approach alongside technical expertise, ensuring success in this dynamic field.
Ways to develop and hone these skills
Developing skills in data analysis and computational science requires a hands-on approach. Start by engaging with online platforms that offer interactive courses. Websites like Coursera, Udacity, and edX provide structured learning paths tailored to your pace.
Join coding boot camps or workshops to immerse yourself in real-world scenarios. These programs often facilitate networking opportunities with industry professionals who can share valuable insights.
Practice is crucial. Work on personal projects or contribute to open-source initiatives on GitHub. This not only helps you apply what you learn but also builds a portfolio that showcases your capabilities.
Participate in hackathons or data challenges on sites like Kaggle. Competing against peers sharpens your problem-solving skills while exposing you to diverse methodologies.
Lastly, find mentorship within professional communities or forums dedicated to data analysis and computational science. Learning from experienced individuals can provide guidance and accelerate your growth in this dynamic field.
Resources for learning and practicing data analysis and computational science skills
The digital age offers a wealth of resources for aspiring data analysts and computational scientists. Online platforms like Coursera, edX, and Udacity provide courses from top universities. These structured programs often include hands-on projects to reinforce learning.
For those who prefer self-study, websites such as Kaggle offer datasets along with competitions that challenge your skills. Engaging in these challenges can sharpen analytical abilities while connecting you with a community of like-minded individuals.
Books also remain invaluable. Titles like “Python for Data Analysis” by Wes McKinney or “Deep Learning” by Ian Goodfellow are essential reads for deepening your knowledge base.
YouTube channels dedicated to data science tutorials can make complex topics more digestible. Visual content is particularly beneficial when grasping statistical concepts or coding techniques.
Joining local meetups or online forums can facilitate networking opportunities and peer support, making the journey less solitary while enhancing skill development through collaboration.
How to showcase these skills on a resume or during a job interview
Highlighting your skills in Data Analysis and Computational Science on a resume requires precision. Use specific examples to demonstrate your expertise. Instead of listing programming languages, mention projects where you utilized Python or R effectively.
Quantify achievements when possible. For instance, say you improved data processing speed by 30% through optimized algorithms. This shows potential employers the tangible impact you’ve made.
During interviews, be prepared to discuss both technical and non-technical skills. Share scenarios that illustrate your problem-solving abilities or critical thinking processes.
Practice articulating complex concepts simply; communication is key in this field. Show enthusiasm for continuous learning too—mention recent courses or certifications relevant to data analysis trends.
Remember, confidence matters as much as content. Approach questions with a positive mindset and showcase not just what you know but how eager you are to apply it in real-world situations.
Advancements and trends in the field that may require additional or evolving skills
The landscape of Data Analysis and Computational Science is constantly changing. Emerging technologies like artificial intelligence and big data are reshaping the industry. Professionals must adapt to stay relevant.
Data privacy concerns and regulations are also on the rise. Understanding compliance requirements can set you apart in this field. Knowledge of ethical data practices is becoming essential.
Cloud computing is transforming how data is stored and analyzed. Familiarity with platforms such as AWS or Google Cloud can enhance your skill set significantly.
Additionally, interdisciplinary approaches are gaining traction. Collaborating with experts from fields like biology or finance requires a blend of domain knowledge along with technical skills.
Keeping an eye on trends in automation tools will be crucial too. As tasks become automated, learning to leverage these tools effectively will give you an edge in Data Analysis and Computational Science jobs.
Conclusion
In an ever-growing world of big data and technology, possessing essential skills in data analysis and computational science is crucial for success. As we continue to advance and evolve with new technologies, it is important to continuously improve and adapt these skills in order to stay competitive in the job market. By incorporating these tips into your learning approach, you can set yourself apart from others and pave the way for a successful career in this rapidly expanding field. With determination, dedication, and continuous self-improvement, there’s no limit to what you can achieve as a data analyst or computational scientist.